Hugging Face facilitates AI accessibility with Meilisearch
Meilisearch powers the discovery of 300,000+ AI models, datasets, and demos in the Hugging Face repository.
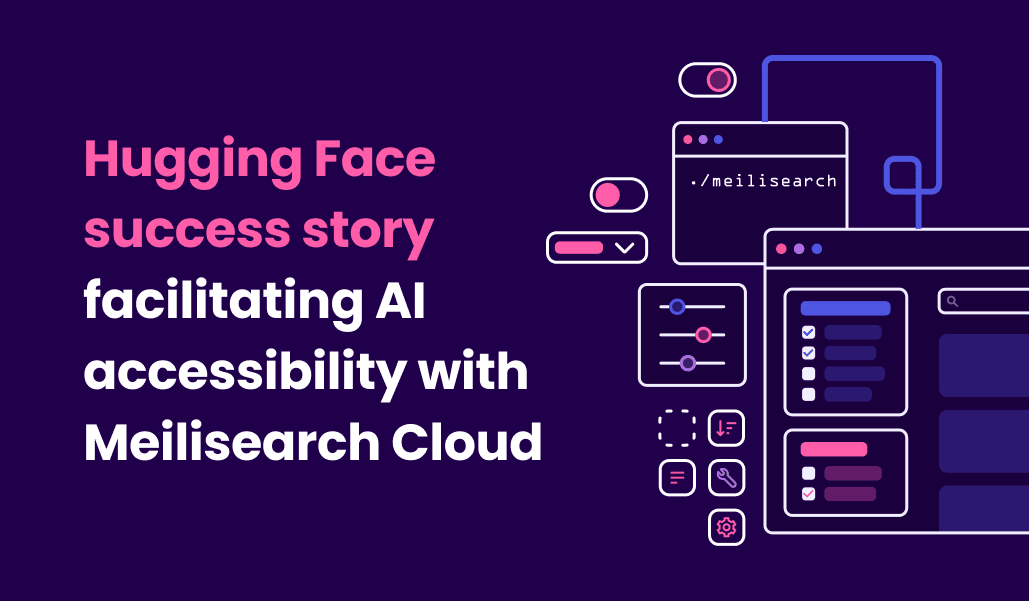
Headquartered in New York and Paris, Hugging Face is an open-source provider of machine learning technologies, allowing users to train, deploy, and share AI models using Hugging Face open-source libraries and the Hub.
By partnering with Meilisearch Cloud, Hugging Face actively fulfills its visionary commitment to advancing the democratization of AI.
“Today, Meilisearch is used by Hugging Face to power the discoverability of 300,000+ AI models, datasets, and demos. It’s really important for AI democratization because if the knowledge is out there, but you can’t access it - what’s the point?” - Mishig Davaadorj, software engineer at Hugging Face
Challenge
On its platform, Hugging Face enables users to upload their AI models, datasets, and demos. With a vibrant community of users constantly engaging with the Hugging Face Hub - a platform for sharing and searching for Machine Learning artifacts - the question of discoverability takes center stage.
The Hugging Face Hub hosts over 220,000 AI models catering to a variety of machine-learning tasks, all neatly stored in repositories. These model repositories are designed to make the exploration and utilization of models as seamless as possible. Each AI model in the Hugging Face repository is accompanied by a model card, a project file containing valuable metadata, which plays a crucial role in enhancing discoverability, reproducibility, and sharing. Model cards also provide important information about AI models’ biases and limitations, model descriptions, and training guidelines, and serve as comprehensive guides for users seeking models on the Hub or uploading their own.
Before the introduction of Meilsearch, Hugging Face relied on a simple filtering and keyword search solution. However, it became evident that there was a growing demand for a more flexible and typo-tolerant full-text search solution. This solution needed to ensure higher default relevancy for every search query and leverage the additional attributes and metadata stored within model cards.
Why Hugging Face chose Meilisearch Cloud
Before integrating Meilisearch into its ML model repository, Hugging Face had already been utilizing Meilisearch's free-of-charge open-source solution as a search engine for its open-source libraries’ documentation, including transformers & diffusers. This documentation comprised approximately 500 pages and had been in use for over a year. Because the team had already gained positive experiences from implementing and operating Meilisearch, when the need for enhanced discoverability in model card searches arose, there was no requirement for additional testing or proof of concept.
1. Customizability of the ranking rules
During the evaluation process, the Hugging Face team also considered Mongo Atlas Search. However, they weren't satisfied with the customizations available for ranking. Several factors were taken into account in order to enhance model card discoverability, such as project names, descriptions, and the number of likes or downloads on each card. Meilisearch has demonstrated greater flexibility and adaptability in accommodating these search criteria.
2. Transitioning to Meilisearh Cloud for its ease of use
Having achieved successful implementation of Meilisearch in its documentation, the Hugging Face team smoothly transitioned to incorporating Meilisearch Cloud into their model cards repository, benefiting from out-of-the-box relevancy.
3. Quality of support and infrastructure outsourcing
The Hugging Face team chose Meilisearch Cloud, in part due to its dedicated support capabilities. Given that achieving highly relevant search results within the repositories is of utmost importance to Hugging Face, the decision to opt for the Cloud version was further influenced by entrusting the infrastructure to the expertise of Meilisearch Cloud specialists. Outsourcing the infrastructure allows the Hugging Face team to enhance their development speed.
Implementation
With Meilisearch already implemented for Hugging Face documentation, the expansion of the search solution to other use cases was smooth, once the ranking and internal rules were in place.
Today, the Meilisearch engine powers the discovery of 220,000 model cards, 38,000 datasets, and 60,000 demos in the Hugging Face repository. The keyword filtering mechanism has already been implemented on the Hugging Face homepage for users who are already familiar with specific model names.
However, with the implementation of Meilisearch, users are given the option to perform full-text searches as well. This search functionality operates not only on the model names and IDs but also includes the entire content of model cards.
The additional button to “Try full-text search” was implemented in order to minimize changes in the search behavior, where users are free to choose the search experience that most fits their preference.
Vision
With the ongoing evolution of AI, Hugging Face anticipates a shift in search behavior and the methods people use to find the projects they need. Hugging Face is looking forward to incorporating Semantic Search into its documentation and model card searches. Currently, the platform is actively testing Meilisearch [VectorDB](/blog/langchain-semantic-search-tutorial/ as one of the potential solutions.
Hugging Face also anticipates gaining more detailed usage insights, encompassing information about users' daily search patterns and the level of activity certain model card projects receive over time.