Enterprise search: a comprehensive guide
Learn how enterprise search transforms data access and boosts productivity. Get expert insights on implementation, providers, and ROI in this guide.
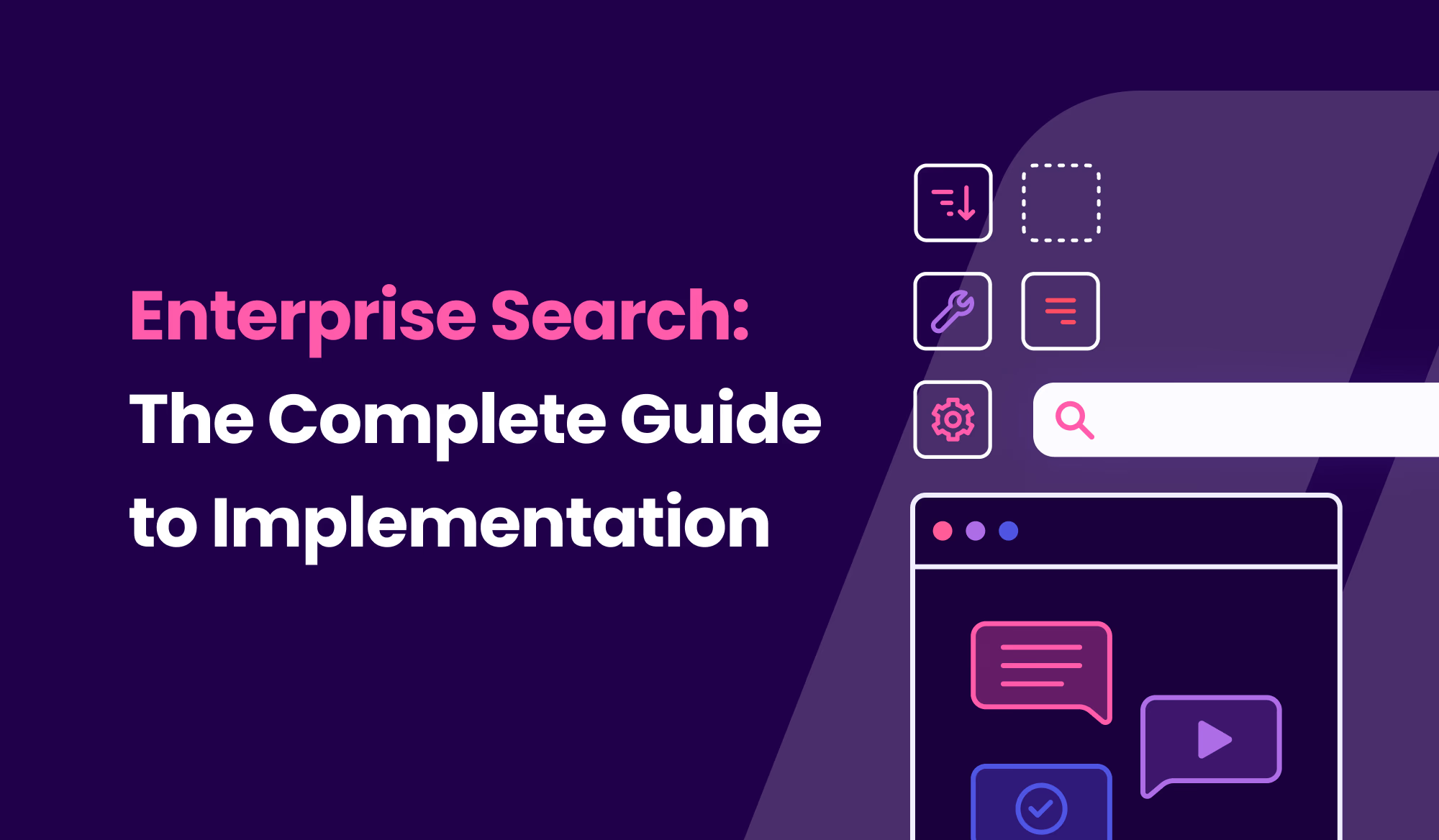
Employees spend an average of 1.8 hours per day searching for information across scattered systems and databases.
Enterprise search transforms this frustrating experience into a seamless journey, helping organizations use their data assets more effectively. This guide will cover everything you need to know about implementing enterprise search.
We'll discuss its core functionality, compare leading providers, leverage AI capabilities, and measure ROI. Whether you're a business leader exploring solutions or a technical professional tasked with implementation, you'll find practical insights to make informed decisions about this critical technology.
What is enterprise search?
Enterprise search is a transformative technology that helps organizations find, access, and use their internal information effectively. Unlike regular web search engines, enterprise search systems handle the complex data landscape within organizations.
Definition of enterprise search
Enterprise search is specialized software that enables organizations to search and retrieve information from various internal systems and data sources.
It goes beyond simple keyword matching by using advanced technologies like artificial intelligence and natural language processing (NLP) to understand user intent and context.
Modern enterprise search solutions handle both structured data and unstructured content (such as documents, emails, and media files).
Importance and purpose of enterprise search
The primary purpose of enterprise search is to boost organizational efficiency and knowledge sharing.
Enterprise search addresses this challenge by providing quick access to relevant data, which improves productivity and decision-making.
A well-implemented enterprise search solution serves critical purposes:
- Helps maintain competitive advantage by ensuring employees can quickly find and leverage institutional knowledge.
- Supports compliance efforts by making it easier to track and retrieve required documentation.
Differentiation from web search engines
Enterprise search differs fundamentally from web search engines. While web search engines like Google focus on publicly available internet content, enterprise search systems target private organizational data. They incorporate sophisticated security controls to ensure users can only access authorized information.
These systems understand your organization's specific context and terminology. For instance, Meilisearch's enterprise solution can be customized to recognize industry-specific terms and acronyms, making searches more relevant.
Benefits of implementing enterprise search
Enterprise search transforms how organizations access and utilize their information assets. When properly implemented, it delivers measurable improvements across multiple business dimensions, from daily operations to strategic decision-making.
Improved internal efficiency
Enterprise search dramatically reduces the time employees spend looking for information. Modern enterprise search solutions cut this time significantly. Teams can quickly locate documents and resources across multiple repositories without switching between systems.
For example, ibuyers.app demonstrates how enterprise search can transform information retrieval by acting like a dedicated research assistant, helping organizations swiftly navigate complex data landscapes and make more informed decisions.
Enhanced customer satisfaction
When customers can easily find what they're looking for, satisfaction levels improve. Enterprise search enhances customer experience in several ways. Support teams access accurate information faster, reducing response times. Self-service portals become more effective, allowing customers to find answers without contacting support.
Better data utilization and decision-making
Enterprise search transforms raw data into actionable insights. Modern solutions don't just find documents—they uncover patterns and connections that drive better decision-making. Teams can discover trends, identify knowledge gaps, and make data-driven decisions more effectively.
Cost-benefit analysis of enterprise search implementation
While enterprise search requires an initial investment, the returns often justify the costs. Organizations typically see ROI through:
- Reduced labor costs from faster information retrieval
- Lower training expenses due to improved knowledge sharing
- Decreased customer support costs through better self-service
- Increased revenue from improved customer experience
How enterprise search works
Enterprise search is more complex than traditional web search engines, involving multiple phases to deliver accurate results. Let's explore how these systems process and retrieve information across an organization's various data sources.
Exploration phase - data gathering and extraction
The exploration phase begins with data discovery and collection across multiple sources. Enterprise search engines use specialized connectors to crawl through databases, document management systems, and digital repositories.
For example, the system might connect to SharePoint for documents, Salesforce for customer data, and internal wikis for knowledge base articles.
During this phase, the search engine identifies and extracts both structured data (like database entries) and unstructured content (such as emails and documents). The system maintains data lineage and tracks metadata, ensuring information remains traceable and up-to-date.
Indexing phase - organizing and structuring data
Once data is gathered, the indexing phase transforms raw information into searchable content. The system analyzes and enriches the data by identifying relationships between different pieces of information. Modern enterprise search solutions like Meilisearch use advanced techniques:
- Creating inverted indexes for quick keyword lookups
- Generating vector embeddings for semantic understanding
- Building relationship maps between related content
- Establishing hierarchical structures for better organization
This phase is crucial for enabling fast and accurate search results. The system continuously updates these indexes as new information is added or modified.
Query phase - retrieving data for users
When users enter a search query, the system springs into action to deliver relevant results. The query phase involves multiple steps.
First, the system processes the user's input, accounting for factors like typos and synonyms. Then it matches the query against the indexed content, considering factors such as:
- Keyword relevance and proximity (using fuzzy search for example)
- User permissions and access rights
- Content freshness and popularity
- Previous search patterns and user behavior
Modern enterprise search platforms can return results in milliseconds, even when searching through millions of documents.
Use of AI/ML in improving search results
Artificial intelligence and machine learning have revolutionized enterprise search capabilities. These technologies enhance search accuracy by understanding user intent through natural language processing, even when queries are conversational or imperfect.
Machine learning algorithms improve results by analyzing user interactions and feedback. For instance, if users frequently select a specific document for certain terms, the system learns to rank that document higher for similar queries.
Handling structured and unstructured data
Enterprise search systems use different approaches for structured and unstructured data. For structured data like database records, the system can directly map fields and relationships, enabling precise filtering and sorting based on specific attributes.
Unstructured data requires more sophisticated processing. The system must analyze and extract meaning from free-form text, images, and other content types.
Modern enterprise search platforms use AI-powered techniques to understand context and relationships within unstructured data, making it as searchable as structured content. This hybrid approach ensures users can find all relevant information, regardless of format.
Types of enterprise search
Enterprise search solutions come in several distinct types, each designed to meet different organizational needs and data access requirements. Understanding these types helps organizations choose the right solution for their specific use case.
Siloed search
Siloed search represents the most basic form of enterprise search. Each department or system maintains separate search functionality.
This approach is common in organizations that have grown organically, with different departments adopting their own tools and systems. While simple to implement, siloed search can create information barriers between teams. For example, sales teams might access customer data in their CRM search, while support teams search separately in their ticketing system.
Federated search
Federated search takes a more integrated approach by searching across multiple data sources simultaneously. When a user enters a query, the system sends it to various data sources and combines the results in real-time.
This method is effective for organizations needing to maintain separate databases for security or compliance reasons. A typical implementation might search across document management systems, email servers, and internal wikis, presenting unified results to users.
Unified search
Unified search creates a single, comprehensive index of all organizational data, regardless of its original source. This approach offers the fastest search performance since all content is pre-indexed in one location.
Modern unified search solutions can process both structured and unstructured data, making it easier to find information across different content types. For instance, an employee could search for a product name and see results from product specifications, customer feedback, and internal documentation in one view.
Choosing the right type of enterprise search for your organization
Choosing the right type of enterprise search depends on several key factors:
- Organizations with strict data governance might prefer federated search to maintain separate data repositories.
- Companies prioritizing speed and seamless user experience often benefit from unified search.
- For organizations handling complex queries and large volumes of unstructured data, AI-powered search offers the most sophisticated solution.
Consider your organization's size, security requirements, and user needs when making this decision. Many modern solutions offer hybrid approaches that combine multiple types to provide the best of all worlds.
Key features of enterprise search
Modern enterprise search platforms come equipped with powerful capabilities that transform how organizations find and use their information. Let's explore the essential features that make enterprise search solutions effective.
Data connectors
Data connectors serve as bridges between enterprise search platforms and various data sources. These connectors enable seamless integration with systems such as document management platforms and customer relationship databases. They automatically sync and index content from different sources, ensuring information remains current and accessible.
Modern enterprise search solutions offer extensive connector options supporting both structured and unstructured data sources. These connectors use various protocols to establish codeless connections between original data sources and search indexes, simplifying implementation for IT teams.
Data security and compliance
Security is a cornerstone of enterprise search implementations. Organizations must protect sensitive information while making it accessible to authorized users. Enterprise search platforms incorporate multiple security layers to achieve this balance.
Modern solutions implement role-based access control (RBAC), ensuring users can only access information relevant to their roles. They also provide encryption at rest and in transit, protecting data throughout its lifecycle.
For instance, Meilisearch's enterprise solution offers end-to-end encryption and advanced tenant isolation, meeting strict compliance requirements like SOC 2.
Multitenancy allows multiple users to share infrastructure with strict data isolation and personalized access controls. Each tenant's data remains separate, ensuring security and individualized experiences within shared platforms.
Analytics and insights
Analytics capabilities help organizations understand how users interact with their search functionality. These insights drive improvements in search relevance and user experience. Enterprise search platforms collect and analyze data about search patterns, popular queries, and failed searches.
Modern analytics tools go beyond basic metrics, offering AI-driven insights that identify trends and patterns in search behavior. This information helps organizations optimize their content strategy and improve search effectiveness over time.
Deployment flexibility
Organizations need search solutions that adapt to their infrastructure requirements. Enterprise search platforms offer various deployment options to meet these needs. Whether cloud-based, on-premises, or hybrid, these solutions provide flexibility in how organizations implement and scale their search capabilities.
Cloud deployments offer advantages like automatic updates and simplified maintenance. On-premises installations provide maximum control over data and security. Some organizations opt for hybrid approaches to balance these benefits. For example, Meilisearch supports both cloud and self-hosted deployments, allowing organizations to choose the option that best fits their needs.
Customizable search UI
A well-designed search interface significantly impacts user adoption and satisfaction. Enterprise search platforms offer customizable user interfaces that organizations can tailor to their specific needs and branding requirements.
These interfaces support features like faceted search, filters, and sorting options, helping users quickly narrow down results.
Scale your search seamlessly from startup to enterprise. Try Meilisearch Cloud and leverage a solution that grows dynamically with your business needs.
Comparing enterprise search providers
The enterprise search market offers several robust solutions, each with distinct strengths and approaches. Let's examine the major players to help you determine which might best suit your organization's needs.
Overview of Meilisearch
Meilisearch stands out as a modern, developer-friendly solution in the enterprise search landscape. It delivers lightning-fast search results in under 50 milliseconds, making it ideal for real-time search experiences.
The platform offers enterprise-grade features like SOC 2 compliance, end-to-end encryption, and advanced tenant isolation. It maintains cost efficiency with an average 50% reduction in expenses compared to alternatives.
Want to see how a real-world enterprise transformed their search experience? Check out the Meilisearch case study with OCTO Technology to learn how they achieved superior search performance and seamless CMS integration.
Overview of major providers
Each major provider brings unique capabilities to enterprise search:
- Elastic Enterprise Search: Excels in flexibility and scalability, offering powerful APIs and advanced search features.
- Microsoft Graph/Copilot: Leverages deep integration with Microsoft 365 apps, providing AI-driven insights and automation.
- IBM Watson Discovery: Stands out for its natural language processing capabilities and enterprise-grade security measures.
Detailed feature comparison
Let's examine how these solutions compare in key areas:
Feature | Elastic Enterprise Search | Microsoft Graph/Copilot | Algolia |
---|---|---|---|
Search Capabilities | Full-text search, vector search, type-ahead | Natural language queries, AI-driven insights | Typo-tolerance, synonyms, geo-awareness |
AI Integration | Anomaly detection, forecasting | AI-powered suggestions | Personalization features |
Scalability | Horizontal clustering | Microsoft ecosystem integration | High-traffic optimization |
Security | Role-based access, field-level security | Enterprise-grade infrastructure | Secure implementation |
Pricing and cost evaluations
Enterprise search solutions offer various pricing models to suit different needs:
- Elastic Enterprise Search starts at $95/month for standard features, without computing costs.
- Microsoft Graph/Copilot requires a $30/user/month add-on to existing Microsoft 365 licenses.
- Algolia uses a pay-as-you-go model at $0.40 per 1,000 records stored and $0.50 per 1,000 search requests.
Organizations should consider both immediate costs and long-term scalability when making their choice.
Strengths and weaknesses of leading enterprise search providers
Each provider has distinct advantages and limitations:
- Elastic Enterprise Search offers excellent flexibility but can become complex to manage at scale.
- Microsoft Graph/Copilot provides seamless integration with Microsoft tools but requires existing Microsoft 365 subscriptions.
- Algolia delivers strong performance but costs can escalate with high search volumes.
- Meilisearch balances these concerns by offering enterprise features with cost efficiency and simpler implementation.
AI and modern capabilities in enterprise search
Enterprise search has evolved significantly with the integration of artificial intelligence and machine learning technologies. These advancements have transformed how organizations discover, access, and utilize their information assets.
Natural language processing
Natural language processing makes enterprise search more intuitive and human-friendly. Instead of requiring exact keyword matches, NLP allows users to phrase queries as they would in normal conversation. This technology understands context, intent, and variations in language.
Modern enterprise search platforms use NLP to handle complex queries like "Show me all projects completed by the marketing team last quarter" or "Find documents about customer retention strategies from 2023." This capability is valuable in organizations where employees speak different languages or use varying terminology for the same concepts.
AI-powered search
AI-powered search represents the latest evolution in enterprise search technology. These systems incorporate machine learning and natural language processing (NLP) to understand user intent and context.
They learn from user behavior, automatically categorize content, and even predict what users might need before searching. For example, an AI-powered search might recognize that when marketing team members search for "campaign results," they're looking for analytics data, while support team members might seek customer feedback reports.
Use of vector databases
Vector databases have become essential components of modern enterprise search systems. They enable semantic search capabilities by converting text, images, and other content into mathematical representations that capture meaning and relationships.
These databases excel at finding similar content even when exact keyword matches don't exist.
For example, Meilisearch's vector search capabilities allow organizations to implement hybrid search experiences that combine traditional keyword matching with semantic understanding. This helps users find relevant content even when their search terms differ from the exact wording in documents.
Retrieval augmented generation (RAG)
RAG represents the latest advancement in enterprise search technology, combining the power of large language models with precise information retrieval. This approach ensures that AI-generated responses are grounded in accurate, organization-specific data.
When users submit queries, RAG systems first retrieve relevant documents from the organization's knowledge base. Then, they use this information to generate contextually appropriate responses.
For instance, if an employee asks about the company's vacation policy, the system can pull information from HR documents and provide a concise, accurate answer while maintaining the ability to cite sources.
This technology is valuable for:
- Creating automated customer support responses
- Generating accurate summaries of long documents
- Providing contextual answers to complex business questions
- Maintaining compliance by ensuring responses are based on approved content
The integration of these AI capabilities has made enterprise search more powerful and user-friendly than ever before. Organizations using modern solutions can leverage these technologies to improve information discovery and knowledge sharing across their operations.
Challenges in implementing enterprise search solutions
Implementing enterprise search comes with several significant hurdles. Understanding these challenges helps teams prepare strategies and avoid common pitfalls during implementation.
Data fragmentation and integration
Data silos and fragmentation present major obstacles for enterprise search implementations. Organizations typically store information across multiple systems, databases, and formats. This scattered data landscape makes it challenging to create a unified search experience.
Many companies struggle with connecting legacy systems not designed for modern integration. For example, older document management systems might lack proper APIs, while cloud-based tools use different authentication methods.
Security risks and compliance
Security concerns often emerge as a critical challenge. Organizations must balance making information discoverable while protecting sensitive data from unauthorized access.
Compliance requirements like GDPR and industry-specific regulations add another layer of complexity. Search systems need to enforce role-based access controls and maintain audit trails of data access. They must also ensure proper data handling across different geographical regions, especially for multinational companies.
Search query complexity
Users expect search systems to understand natural language queries and complex search intentions. However, implementing these capabilities presents significant technical challenges.
Different departments often use varying terminology for the same concepts, making it difficult to deliver consistent results. For instance, HR might refer to "personnel files" while legal uses "employee records."
Modern enterprise search platforms tackle this through features like synonym management and natural language processing, though configuring these correctly requires effort.
High costs and ROI calculation
The financial investment in enterprise search can be substantial, with costs spanning software licenses, infrastructure, and ongoing maintenance. Organizations often struggle to justify these expenses, especially when immediate returns aren't easily measurable.
Implementation costs frequently exceed initial estimates due to unforeseen complexity in data migration and system integration.
Companies need to consider direct costs (like software licenses and hardware) and indirect costs (such as staff training and productivity dips during transition). A thorough ROI analysis should account for improved productivity, reduced search time, and better decision-making capabilities.
Common pitfalls in enterprise search implementation and how to overcome them
Many organizations fall into predictable traps when implementing enterprise search. One common mistake is underestimating the importance of content quality and metadata management. Without proper data cleanup and standardization, even the most sophisticated search engine will struggle to deliver relevant results.
Another frequent pitfall is insufficient user training and change management. Organizations often focus on technical aspects while neglecting user adoption strategies. Success requires a balanced approach that includes comprehensive user training, clear communication about system benefits, and regular feedback collection for continuous improvement.
To overcome these challenges, companies should start with a pilot project in a single department or use case. This approach allows teams to identify and resolve issues before rolling out enterprise-wide.
Why enterprise search matters for business success
As businesses generate massive amounts of data, implementing a robust enterprise search solution becomes a strategic imperative for maintaining competitive advantage.
By carefully evaluating your needs, choosing the right provider, and following implementation best practices, you can transform your organization's ability to discover, access, and leverage critical information across all your digital touchpoints.