Beyond the hype: practical AI search strategies that deliver ROI
Unlock the power of AI-powered search for your SaaS business. Learn key features, budgeting tips, and implementation strategies to boost user engagement
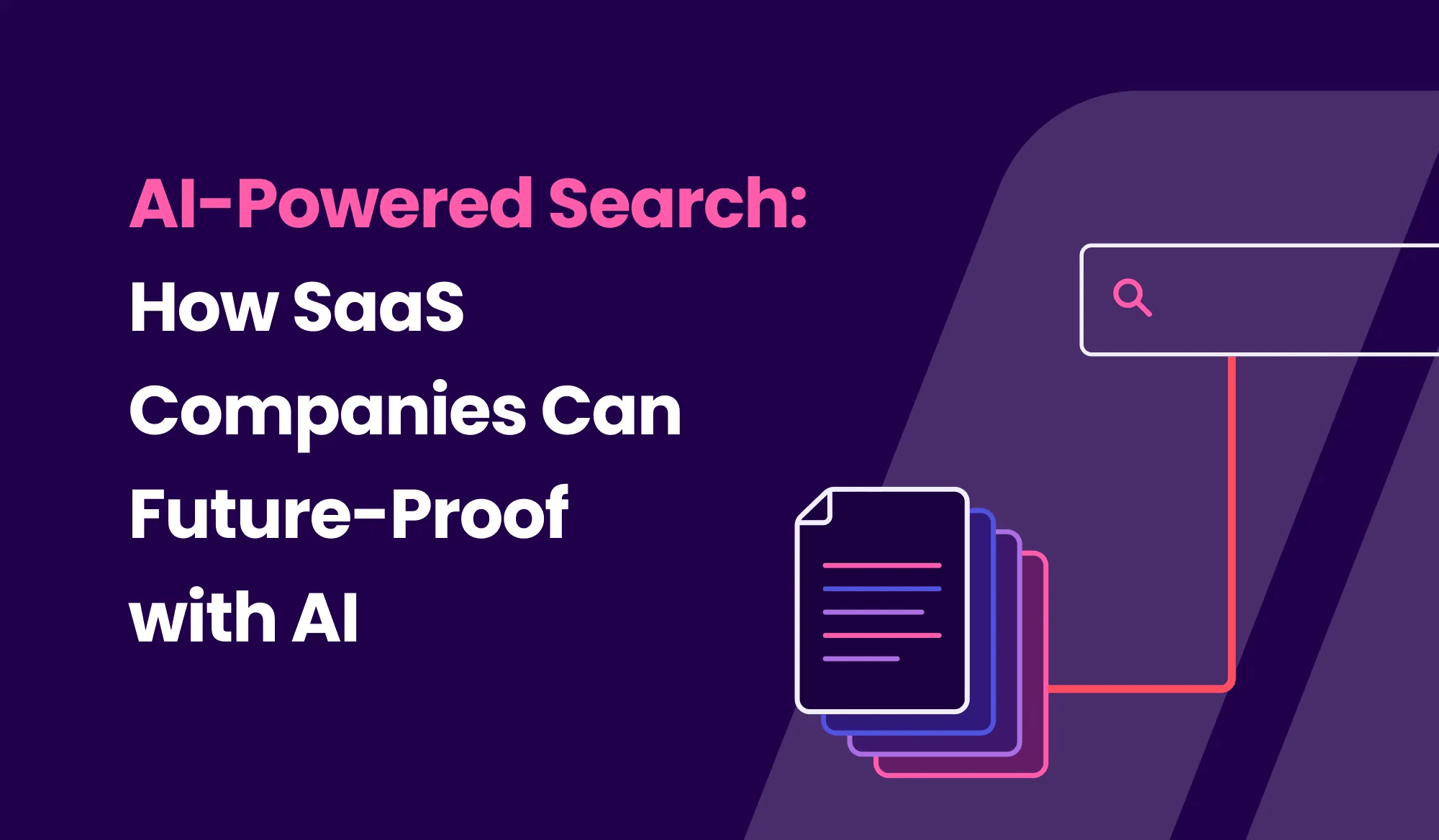
Imagine this: You're a SaaS product manager who just left a board meeting where the CEO announced, "We need to invest in AI search!" The budget is set, the engineering team is eager, and time is running out. But as you explore AI-powered search, the reality strikes hard. The field is filled with buzzwords, big promises, and high costs. You start to question, "Will this really deliver the ROI we need?"
The problem is an unrealistic outlook, wrong metrics, and the "more is better" mentality regarding features. SaaS companies spend millions on AI search, only to deal with rising costs, unhappy users, and doubts about their approach.
But there's good news. With a pragmatic, business-focused approach, you can use AI search to achieve real results. This can be done without overspending or losing focus.
This article will cover crucial strategies for implementing AI-powered search effectively. Topics include budgeting, feature selection, measuring success, and future-proofing your SaaS. Prepare to move beyond the hype and truly harness AI search.
Core features of new generation AI-powered search
Demystifying AI search technologies
AI-powered search represents a significant leap forward from traditional keyword matching. It employs sophisticated algorithms that understand context and user intent.
At its core, this technology combines machine learning and natural language processing to interpret queries as humans naturally express them. For instance, when Bookshop.org implemented AI search, their system understood complex queries about books. It accounted for variations in titles, author names, and even thematic searches that traditional systems would struggle with.
Storage layer
Vector database
Vector databases form the foundation of modern AI search systems. They store complex data representations that capture semantic relationships between items. Unlike traditional databases, these specialized systems can efficiently process high-dimensional data. This enables lightning-fast similarity searches across millions of items.
Multiple DBs for full-text search
The architecture of modern search systems often employs multiple specialized databases features working together. These include prefix databases for instant search suggestions, inverted indexes for rapid full-text search, and specialized stores for handling typos and variations.
Capabilities layer
Full-text search
Full-text search remains a cornerstone of modern search systems. It provides precise lexical matching with advanced features like typo tolerance and prefix searching. This capability is especially valuable in e-commerce scenarios. Users often search for specific product codes or exact phrases.
Vector search
Vector search enables semantic understanding by converting search queries and content into mathematical representations that capture meaning, not just keywords. This technology powers the ability to find relevant results even when queries don't exactly match the target content. It was transformative for Bookshop.org's vast catalog of six million books.
Hybrid search
Hybrid search combines the precision of full-text search with the semantic understanding of vector search. It offers the best of both worlds. This approach allows systems to handle both precise queries and more exploratory searches effectively. It delivers relevant results regardless of how users express their needs.
Application layer
The application layer transforms raw search capabilities into practical features that drive business value. It includes personalization systems that adapt results based on user behavior, semantic search that understands natural language queries, and recommendation engines that suggest related items.
Hugging Face leverages this layer to help users discover relevant AI models based on specific use cases and preferences. Similarly, HitPay uses it to enable merchants to prioritize specific products in search results based on business rules.
Want to dive deeper? Check out our detailed comparison of full-text vs vector search to understand their unique strengths and use cases.
Use cases that demonstrate tangible benefits
AI-powered search is transforming how users interact with digital platforms beyond the traditional search bar.
Bookshop.org's implementation demonstrates a compelling impact: their conversion rate jumped from 14% to 20% after switching to AI-powered search, representing a 43% increase in search-to-purchase conversion. This improvement came from the system's ability to understand complex book queries and handle their massive six-million-item catalog effectively.
For Hugging Face, AI search solved the challenge of making 220,000 AI models, 38,000 datasets, and 60,000 demos discoverable to their technical audience. Their implementation goes beyond simple keyword matching, allowing developers to find relevant models based on use cases, performance characteristics, and technical requirements. The system's ability to understand technical context and relationships between different AI models has made it significantly easier for users to find the right tools for their specific needs.
HitPay's experience highlights how AI search can bridge the gap between online and offline retail operations. Their point-of-sale system uses AI search to help sales assistants quickly locate specific products across multiple locations, while simultaneously powering their e-commerce storefronts. The system's ability to sync product catalogs across channels and prioritize frequently accessed items has resulted in a 50% increase in search API response speed. This directly improves the customer experience both in-store and online.
Budgeting for AI-powered search
Determining AI budget allocation
Enterprises are rapidly adopting generative AI, dedicating upwards of 10% of their IT budgets by 2025. You can allocate between 5-15% of IT budgets to AI initiatives. the ideal investment varies based on company size, sector, and goals.
When budgeting for AI-powered search integration, consider these factors:
- The scale of your existing search infrastructure
- The complexity of your data and search requirements
- The size of your user base and expected growth
- Your team's existing AI and machine learning expertise
- The level of customization you require
Breakdown of costs: R&D, implementation, ongoing optimization
The true cost of AI search implementation
Implementing AI-powered search requires careful consideration of both direct and indirect costs. The initial investment covers infrastructure setup, data preparation, and integration costs.
For example, Bookshop.org's transition from Elasticsearch to a more sophisticated AI search solution involved not only technology costs but also resources to properly index and configure their six-million-item catalog. Companies must also factor in ongoing costs for maintenance, updates, and infrastructure scaling as search volume grows.
Check out our analysis of Meilisearch vs. Elasticsearch, for a detailed comparison of the two search solutions.
HitPay demonstrates this approach â they began with an open-source solution to validate their use case before transitioning to a managed cloud service. This allowed them to optimize costs while ensuring reliability for their business-critical search functions.
Avoiding budget black holes
The most significant hidden costs often stem from unexpected infrastructure scaling needs and ongoing optimization requirements. Companies frequently underestimate the resources needed to maintain search relevance and manage growing data volumes.
Infrastructure costs and scalability considerations
Infrastructure is critical for AI-powered search implementation.** Key considerations include:
- Computing power: AI models, especially during training, require substantial computational resources. This may necessitate investment in high-performance hardware or cloud computing services.
- Storage: AI systems need to process and store large amounts of data. Ensure your storage solutions can handle this increased load.
- Network capacity: If your AI search system processes queries in real-time, upgrade your network infrastructure to handle increased traffic.
- Scalability: As your user base grows, your AI search system needs to scale. Cloud-based solutions offer flexibility for scaling but may have higher long-term costs compared to on-premises solutions.
ROI calculation framework for AI search investments
Measuring return on investment requires tracking both direct and indirect impacts of AI search implementation. Key metrics should include:
- Conversion rate improvements (like Bookshop.org's 43% increase)
- Operational efficiency gains (such as HitPay's 50% faster search response times)
- Customer satisfaction metrics
- Reduced support costs from improved self-service capabilities
For SaaS businesses, significant returns often come from improved user engagement and reduced churn. When calculating ROI, companies should consider both immediate performance improvements and long-term benefits, like increased customer lifetime value and reduced operational costs.
KPIs for measuring success
Beyond vanity metrics
Basic metrics like search volume and click-through rates provide some insight, but often mask deeper performance issues. The most telling indicators come from user behavior patterns and business outcomes.
For example, Bookshop.org's success wasn't measured by the number of searches performed, but by the 20% conversion rate from search to purchase. This metric is directly tied to revenue generation.
Decoding search performance that actually matters
Search performance metrics should reflect real user success rates rather than just technical measurements.
Hitpay's 50% improvement in search API response speed impacts in-store customer service and online shopping experiences.
The most telling metrics often combine multiple data points to create a complete picture of search effectiveness. Consider tracking:
- Search-to-conversion ratio: Like Bookshop.org's jump from 14% to 20% in search-driven purchases
- Query refinement rate: How often users need to modify their search terms to find what they need
- Time-to-content: How quickly users find relevant information after initiating a search
- Zero-result rates: The percentage of searches that yield no results, indicating gaps in content or search capability
These metrics should be analyzed in context with your specific business objectives. For a knowledge base, success might mean reduced time-to-answer. For an e-commerce platform, it could mean higher average order values from search-driven purchases.
Warning signs: KPIs that signal AI search failure
Several indicators can reveal problems with your AI search implementation. Watch for:
- High abandonment rates after search attempts
- Increased support tickets related to finding information
- Growing use of alternative navigation methods
- Declining engagement with search-discovered content
When Hugging Face noticed users bypassing their search functionality in favor of direct repository browsing, it signaled a need to improve their search relevance and feature set. Monitoring these warning signs helps identify issues before they impact user satisfaction and business outcomes.
Selecting the right AI search features for your SaaS business
What use cases they enable
AI search features should align with specific business objectives and user needs.
Bookshop.org's implementation demonstrates this principle perfectly. Their focus on semantic understanding and typo tolerance addressed the challenge of helping users find books across a vast catalog, even with imperfect queries. This targeted approach led to their impressive 43% increase in conversion rates.
AI search feature mapping
Semantic search capabilities are valuable for content-rich platforms. Hugging Face's implementation helps users navigate their extensive repository of AI models. Users can find relevant models even when search terms don't exactly match model names or descriptions, significantly improving discovery rates.
Similarity search is particularly useful in e-commerce contexts. HitPay uses this feature to help merchants connect customers with related products. This goes beyond category matching to understand product relationships based on multiple attributes, enhancing cross-selling opportunities.
Personalization features are crucial for platforms with diverse user bases. For instance, HitPay's implementation allows merchants to pin frequently accessed products, automatically adapting search results based on business-specific patterns and preferences.
When more features mean less value
Not every AI search feature delivers equal value for every business case. Hugging Face's experience shows the importance of prioritizing features that align with core user needs. They focused on making their vast technical documentation and model repository discoverable rather than implementing every available search feature.
The key to feature selection lies in understanding user behavior patterns and business objectives. For example, while multi-modal search might seem cutting-edge, Bookshop.org achieved significant results by focusing on fundamental features like semantic understanding and typo tolerance. These features directly addressed their users' primary needs.
Ready to supercharge your search experience? Explore Meilisearch Cloud and deliver lightning-fast search results that will keep your users engaged and boost your conversion rates.
Best practices for implementing AI-powered search
The implementation gauntlet
Successful AI search implementation requires a methodical approach that prioritizes user needs over technical complexity. HitPay's implementation journey is instructive.
They started with a simple internal dashboard search before expanding to customer-facing features. This gradual rollout allowed them to refine their approach with minimal risk. They achieved a 50% improvement in search API response speed while maintaining system stability.
When Bookshop.org transitioned to their new search system, they started with a test environment. This allowed them to validate their approach before full deployment. This methodical strategy enabled them to maintain search performance during their busy holiday season while implementing significant improvements.
Critical decision points in AI search deployment
Key decisions during implementation should focus on:
- Initial feature set selection based on immediate user needs
- Data indexing strategy and update frequency
- Performance monitoring and optimization approaches
- User feedback collection and integration methods
Bookshop.org's team made their ranking rules accessible to non-developers, allowing for quick iterations based on business needs without requiring engineering intervention. This approach enabled them to maintain search relevance during peak periods while continuously improving the user experience.
Future proofing your SaaS with AI-powered search
Cutting through the AI hype cycle
The future of AI-powered search extends beyond current capabilities. Successful implementation requires distinguishing between genuine innovations and marketing hype.
Hugging Face's approach to testing vector database integration for semantic search demonstrates how companies can practically evaluate emerging technologies.
Organizations can evaluate search solutions based on actual business needs. This approach helps avoid chasing trendy technologies that fail to deliver meaningful results.
Stay informed about the latest in search technology, product updates, and industry insights. Subscribe to our newsletter and be the first to know about Meilisearch Cloud's exciting developments.
Strategic long-term AI search planning
Long-term success requires building flexibility into search implementations. HitPay's evolution from basic search functionality to a sophisticated multi-channel solution shows how search capabilities can grow alongside business needs. Their experience highlights the importance of choosing solutions that can scale and adapt as requirements change, rather than being locked into rigid implementations that limit future options.
The most sustainable approaches focus on creating adaptable search infrastructures that can incorporate new capabilities as they mature. Bookshop.org's implementation demonstrates this principle. Their search architecture allows them to continuously refine ranking rules and add new features without disrupting core functionality. This flexibility enabled them to achieve immediate benefits while maintaining the ability to adopt new AI capabilities as they become available.
As AI technologies evolve, AI-powered search has become a critical differentiator for SaaS businesses. Success stories of companies like Bookshop.org, HitPay, and Hugging Face show that thoughtful implementation of AI search capabilities can deliver concrete business results.
These include increased conversion rates and improved user engagement. The key lies in selecting and implementing solutions that align with specific business needs while maintaining the flexibility to adapt as technology and user expectations evolve.
Need a custom search solution tailored to your unique requirements? Schedule a Consultation with our expert team and transform your search strategy.